AI transcription accuracy for contact centres and businesses: An overview
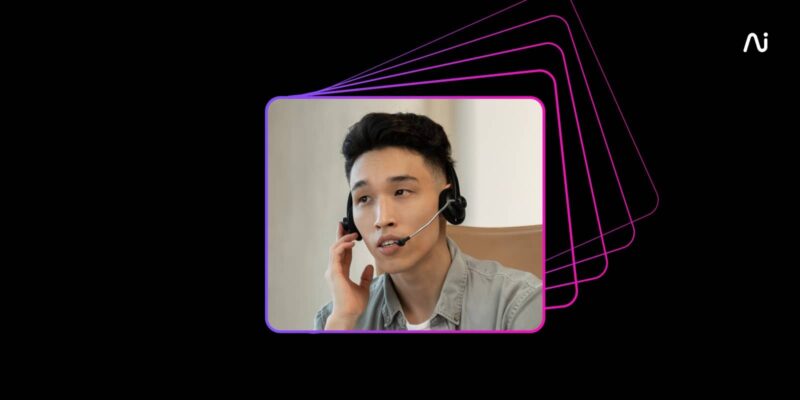
With an increasing push to automate customer service interactions and derive business insights from customer conversations, AI technologies such as Natural Language Processing (NLP) and Automatic Speech Recognition (ASR) have become more and more important in contact centre workflows.
But in order for contact centre AI to be effective from a business perspective—say, at detecting customer sentiment or identifying follow-up tasks mentioned in a call, there’s one essential element: accurate call transcription.
I work with an incredible speech recognition team comprising linguists, AI researchers, and engineers who are the brains behind Dialpad Ai, and in this blog post, we’ll explore why call transcription accuracy is so important for contact centres—and why it’s difficult to achieve.
We’ll dive into different types of insights you can glean from accurate transcripts, and also take a closer look at why simply putting your own branded user interface on a general ASR system is not good enough if you want to achieve a business-specific goal like understanding customer feedback or training sales reps on how to handle objections more effectively.
💡 What is an ASR system?
In a nutshell, an ASR or Automatic Speech Recognition system is a system that decodes an audio signal and turns it into a transcript of the words being spoken.
What does transcription accuracy mean?
Transcription or ASR accuracy is typically measured in terms of “word error rate” (WER), which is the percentage of words that are mistranscribed by the model compared to the ground truth transcript.
💡 “Ground truth transcript”:
The words that were actually said in the conversation. When we train a model via supervised learning, we have matching pairs of audio and transcript. The transcript in there is a verbatim transcript transcribed by a human, which should accurately reflect the words that can be heard in the audio.
A model with a WER of 30% (roughly getting 7 out of every 10 words correct) is generally considered the bare minimum viable for readability—if you needed these transcripts to generate a call summary or track key moments in a call, for example, a higher WER than 30% would be unusable.
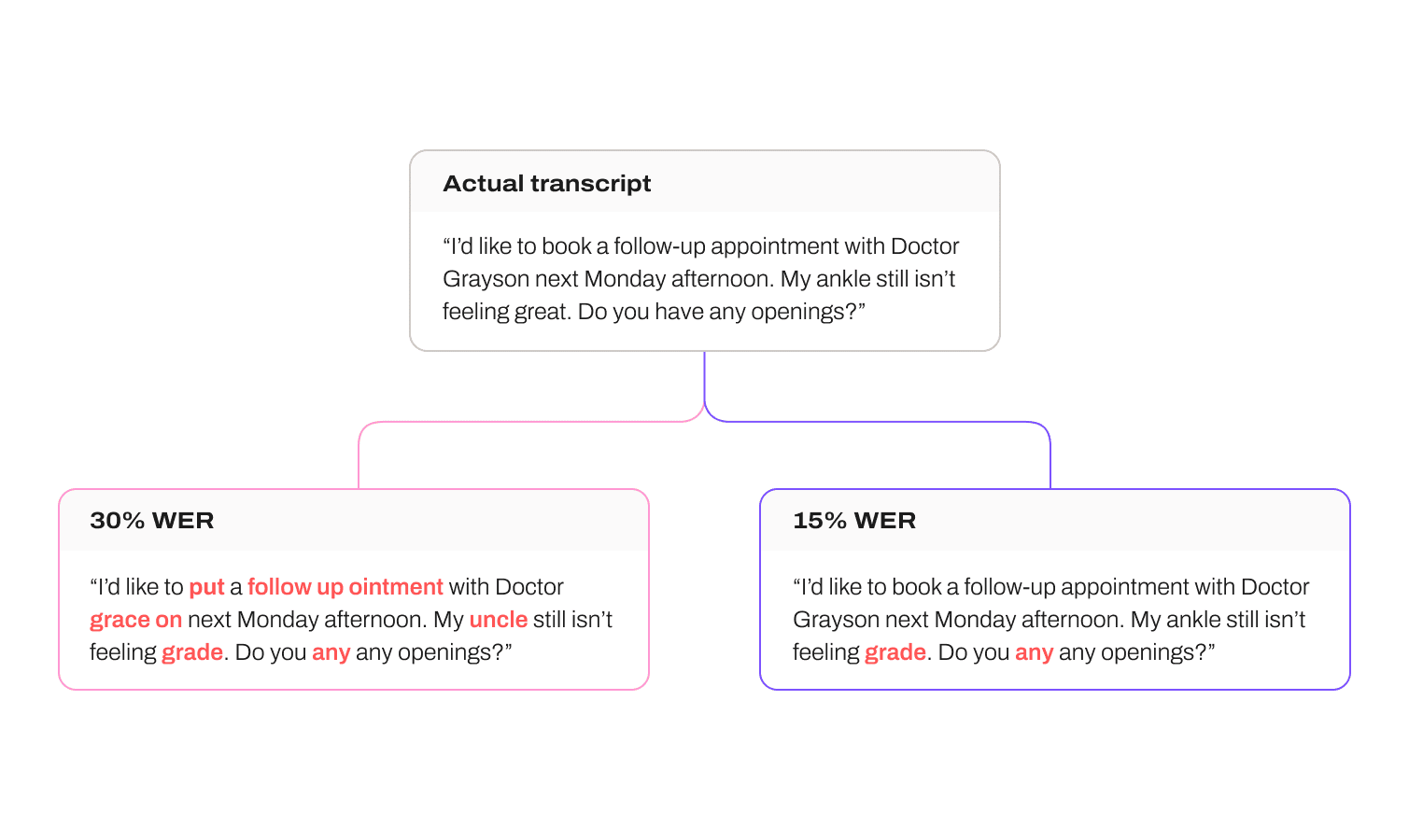
What about model size?
You may have noticed many recent industry press releases around AI tools that mention model size, with companies advertising models with "X million/billion parameters."
While it's true that, all else being equal, bigger models often perform better, what's important to keep in mind is that bigger models also cost more—both in terms of actual compute costs, as well as latency (the time taken to output a model prediction). Here’s an example to show how that would impact a business that’s using an AI tool like this:
Say, your company, Company X, needs a contact centre analytics solution with transcription, and your options are:
- Dialpad,
- Company Foo, who also has an internally developed and hosted ASR model, but is 100x "bigger" in model size, and
- Company Bar, which has slapped its own UI (user interface) on a generic LLM (Large Language Model) like Whisper through an API
In this case, Company Foo has higher operating costs due to their bigger model, and they pass that along to you, their client.
Company Bar pays whatever Whisper charges them per API call, and passes part of those costs along to you, the client.
Dialpad has a smaller, but more efficient model which allows for faster (near real-time) outputs, and has less overhead in terms of compute costs—which benefits you, the client.
Why is transcription accuracy so difficult?
ASR—like most AI systems—is trained via “supervised learning,” which is when you feed some audio, along with a matching transcription, into an AI system. Eventually, the AI will learn which audio patterns correspond to particular bits of text.
These systems are only as good as the data they are trained on, and they tend not to be very good at identifying patterns in data that doesn’t look or sound like the data they’ve been trained on.
Here’s an example:
If I wanted to train an image classification AI system, I’d give it a lot of pictures (which are basically digitized patterns of pixels) along with their corresponding labels.
If I’m training a “cat vs dog” classifier and all of the dog images I have on hand are of labradors, shepherds, and collies, then the system has a good chance of failing if I feed it an image of a chihuahua or a pug, since these don’t look like the images the system was trained on.
👩🔬 Fun fact:
In technical terms, we’d say these are “outside the training distribution.”
The same situation applies in ASR. If I train my system exclusively on clean microphone speech in a noise-free environment, it will be bad at transcribing audio with background noise. If I train it exclusively on high-fidelity audio sampled at 44KHz, it will be bad at transcribing telephone calls (which are sampled at 8Khz).
But this extends beyond properties of the audio, since ASR systems also learn about the patterns in the content, or textual, part of the training data. For example, if I train an ASR system on true crime podcasts, it will probably be bad at recognizing and transcribing business jargon or governmental proceedings, since it has no familiarity with or exposure to those kinds of specialized vocabularies.
In addition to that, the recognition of potentially important keywords (e.g. brand names and technical terms) is difficult because of how rarely they appear in conversation. But these are still important in the context of business communication because they usually highlight the key topics of the conversation and are thus essential to understanding the conversation.
Why transcription accuracy matters
It often seems to be lost among the marketing language and promotional ads, but I can’t overstate the importance of accuracy when it comes to AI-powered transcription, whether it’s a feature in your contact centre software, conversation AI platform, enterprise phone system… You name it.
The reason: it’s the backbone of all the other cool things that AI can do, from live sentiment analysis to keyword and topic tracking, customer insights and agent assists. And we’re still only scratching the surface of what customer service and sales AI can do!
Now, let’s look at a few specific reasons why transcription accuracy is so important.
It helps you provide a good customer experience
One of the most commonly cited benefits of accurate transcripts is that they help businesses improve the customer experience.
If an agent is struggling during a call with a customer, often they’ll message their manager or supervisor to ask for help.
But if that manager could proactively spot when agents are in trouble—say, through real-time transcriptions of all active calls—then they could step in early enough to turn things around, before the customer gets too upset:
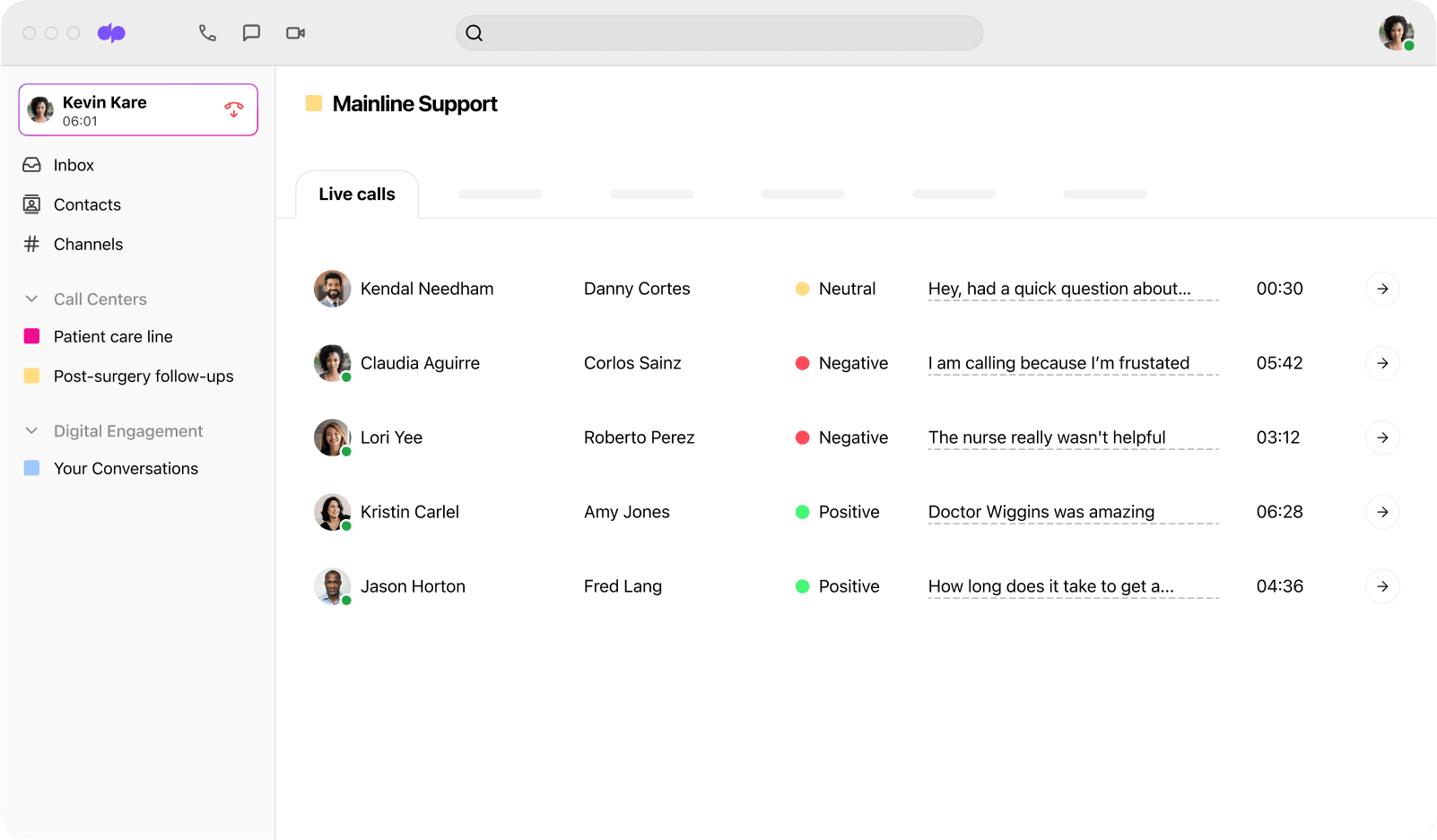
Dialpad Ai can analyze the sentiment of multiple calls in real time
It lets you quickly find insights hidden in customer feedback
Accurate transcripts are helpful after the call too. Not only can business leaders review them to better understand issues and potential opportunities directly from the customer’s perspective, having a transcript (as opposed to just a call recording) means that you can search all of this valuable customer data for the information you need.
For example, which products or services are customers calling about the most this month? Which bugs or troubleshooting questions are coming up most frequently or causing the most problems for agents?
With call recordings, managers would’ve had to listen to each one from beginning to end and take notes, which is extremely time-consuming or even outright impossible, for high-volume contact centres. With a searchable transcript, they can do this much more quickly:
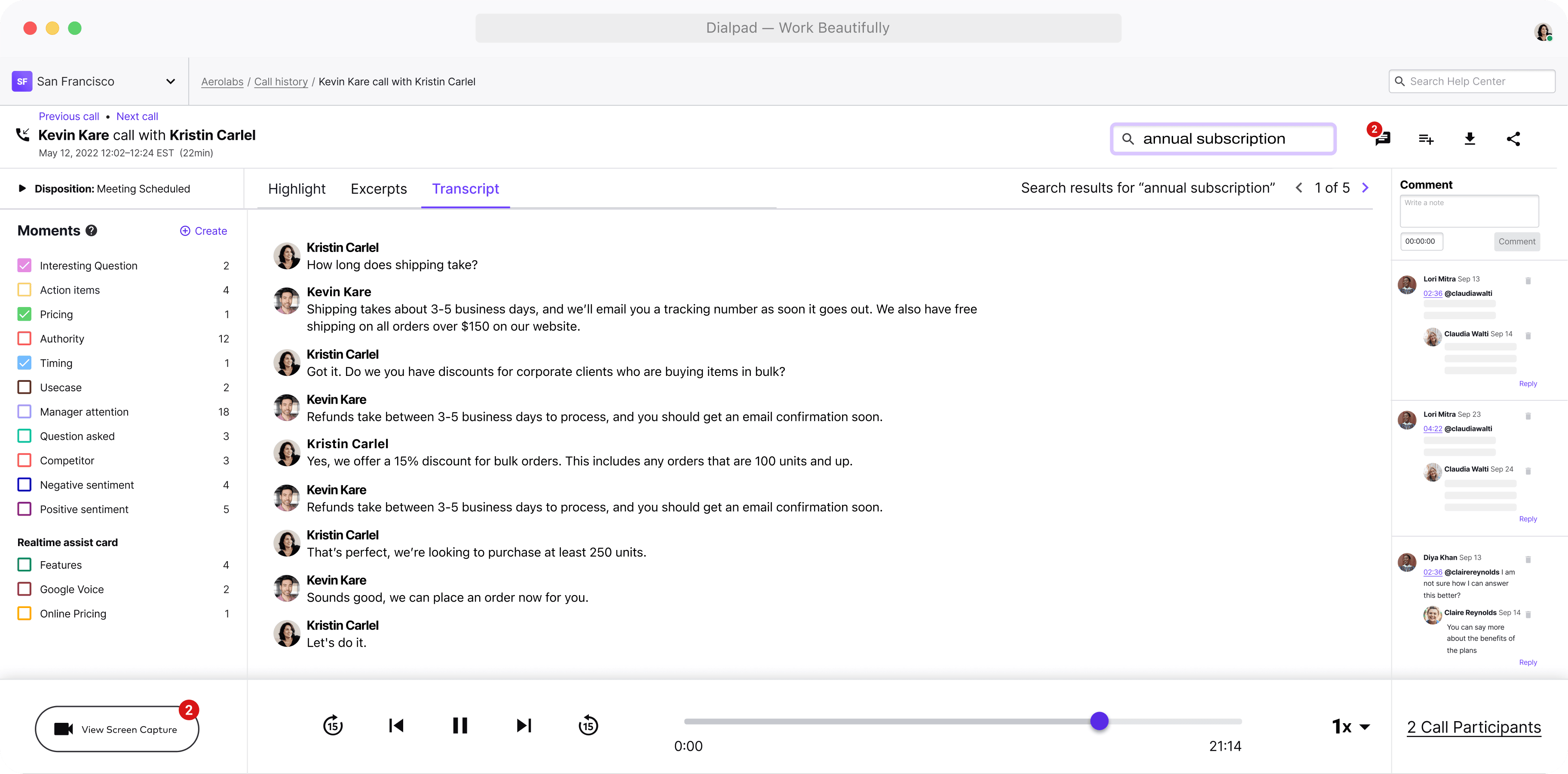
Dialpad’s AI-powered communication solution automatically generates searchable transcripts after every call.
It empowers managers to do faster, better quality assurance
Similarly, this helps customer support and contact centre managers provide better post-call quality assurance and agent training.
Taking the example from the previous item, if a customer support supervisor can see what types of troubleshooting questions their agents are struggling with the most, they can use this insight to improve training materials and provide more targeted coaching.
It helps you maintain compliance
Having accurate transcripts is extremely important for compliance purposes. In industries such as finance and healthcare, companies are required by law to keep records of customer interactions.
These records must be accurate and complete, and any discrepancies can result in fines or legal action.
It allows business leaders to make more accurate forecasts
Outside the contact centre environment, another key use case for accurate transcripts is in helping identify—and predict—customer churn more reliably.
Churn is a major concern for businesses across many industries, and one of the most challenging things about churn is it can be difficult to predict which customers are at risk of leaving. Without this information, you can’t really create a plan of attack to get ahead of things and proactively make improvements to retain customers.
With good transcripts of churn-related customer conversations, business leaders can more easily (and quickly) identify patterns that are associated with churn, such as frequent complaints about certain products, pricing, or other factors.
In all of these cases, transcript accuracy is crucial. The quality of the insights gleaned from customer conversations depends fully on the quality of the transcript. If the transcript contains errors or is incomplete, the resulting analysis will be inaccurate or incomplete as well. In fact, according to McKinsey, incomplete data sets and inaccurate transcripts are two of the three main challenges that businesses face when they try to understand direct customer feedback.
What makes Dialpad Ai different?
Dialpad Ai is unique in a few ways, including the type of datasets it’s trained on and processes that ensure the outputs are 100% focused on business use cases.
But the biggest differentiator is that the datasets that Dialpad Ai is trained on is 100% proprietary, based on anonymized business conversations that happen on Dialpad’s communications platform—which gets more accurate as it learns from more data, and also makes the model more relevant for our customers’ use cases.
These datasets are specialized for our various products (like Dialpad Ai Meetings and Dialpad Ai Contact Centre), as well as different acoustic situations (like low-quality audio), dialects (like Australian English), and languages (like Spanish), among others.
We routinely verify the performance of our models, as well as those of our competitors, against these test sets. (For example, we benchmarked OpenAI’s Whisper against our internal test sets and it performed 10–15% worse in terms of WER.)
💡 Side note:
Note that achieving transcription accuracy is one thing, but doing it in real-time is another. If your business or contact centre team wants to be able to let supervisors monitor call sentiment or coach agents in real time, having the most accurate AI transcription tool won’t necessarily be helpful if it can only do this transcription after the call.
One of the reasons Dialpad’s ASR is so good is because we train our models on real business conversations within the domains of our customers’ industries—which means that general purpose AI systems can’t hope to keep up in terms of transcription accuracy.
🔒 A quick note on data privacy:
We anonymize all data from customer calls when using it to train Dialpad Ai. Our customers always have the option to have Dialpad Ai turned on to help contribute to the accuracy of the system—or to turn it off if they don’t want to make their anonymized data available. Learn more about Dialpad’s security and how we’ve designed Dialpad Ai to help our customers stay compliant.
Dialpad Ai’s ASR vs other ASR systems
So, knowing that Dialpad Ai can transcribe calls accurately—in real time—how does that compare with other ASR systems?
For example, OpenAI's Whisper is an AI system (a core component of which is ASR) that does natural language processing and enables transcription, and OpenAI recently launched an API that lets developers easily wrap Whisper into their own applications and services. On the face of it, these tools combine to dramatically reduce the barrier to entry for businesses that want to launch contact centre AI features.
But these models are generally not good enough to ensure accurate transcripts from a business use case perspective. There are several reasons for this.
First, most of these models aren’t designed specifically for call transcription. While they can be trained on call data, they may not be optimized for the unique challenges of call transcription, such as background noise or low fidelity—remember, telephone calls have inherently lower quality audio than, say, CDs.
Second, these models are not perfect. Like all machine learning models, they’re only as good as the data they are trained on. If the training data isn’t tailored to the use case, then the outputs likely won’t be very helpful. If you work in a car dealership, for instance, you’d want your AI to be trained on a dataset of customer conversations about buying, selling, and fixing vehicles (so it can transcribe automotive terminology accurately).
Long story short: If you want to use AI transcription to help your agents or teams have better business conversations, you’ll need an ASR that is trained on a data set that’s specific to business conversations (instead of just any old audio clips that you can get your hands on).
On a related note, this is the biggest bottleneck for any company that is using AI: acquiring enough data to build a useful model for your specific needs. Yes, consumer-oriented ASR models and many Large Language Models are trained on huge datasets, but often this data is so broad and unfocused that it’s not realistically helpful for businesses.
For example, a business conversation-trained model like Dialpad Ai’s can pick up unique terms (like product names, unusual company names, and other business jargon) much more easily than a general-purpose ASR system.
💡 Did you know:
As of April 2023, Dialpad Ai has processed over four billion minutes, or over 66 million hours of business conversation-focused voice and messaging data.
Are your AI tools giving you the real-time transcription accuracy you need?
With any business-focused AI tool today, transcription accuracy is a key consideration because it is the bedrock of all the other neat AI functionality that sales, customer support, marketing, and other teams find helpful.
Whether you want to forecast revenue more accurately, coach agents in real time, or uncover more customer intelligence, all of this is possible only with accurate AI transcription.
Get a hands-on look at how your team or business can get this with Dialpad Ai!
Get a hands-on look at how Dialpad Ai works
Book a demo with our team, or take a self-guided interactive tour of the app first!